Keynotes
Keynote 1: |
Keynote 2: |
AI-BigData Convergence (ABC)
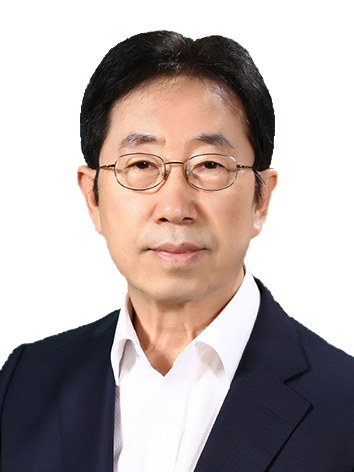
Won Kim
Distinguished Professor and AI Vice President of Gachon University, near Seoul, Korea
Abstract:
In this keynote, I would like to share my vision for the convergence of AI and Big Data, which I will call the “ABC” to make it easy to remember. It is an initiative that can propel the current smart data processing to the next level.
The ABC vision is based on advancing both AI and Big Data through promotion of a fuller use of data, and a symbiotic relationship between AI and Big Data. The fuller use of data means the use of higher quality data and multimodal data for both AI and Big Data. The symbiotic relationship means to advance AI and Big Data by leveraging each other.
The initiative can significantly enhance the performance (both accuracy and speed) of ML/DL; significantly broaden the scope of data science applications, and enhance the productivity in developing such applications; and enhance the quality of data, and lead to a better maintenance of the data to serve as input to ML/DL model training and validation.
This ABC initiative will require participation by a robust segment of the Big Data and ML/DL communities, including researchers, data scientists, application developers, open source developers, and hardware designers.
In this keynote, I will outline an initial scope of the R&D for the ABC Initiative that merits attention from the community.
Bio:
Won Kim is currently a distinguished professor with Software Department at Gachon University, near Seoul, Korea. He is also AI Vice President of Gachon University and is Managing Director of the National Program of Excellence in Software Education at Gachon University, sponsored by the Korean Ministry of Science & Technology and Information Technology.
Before joining the faculty of Gachon University, he was a professor with SKKU (SungKyunKwan University), and a senior vice president of Samsung Electronics, both in Korea.
He was the founder and CEO of UniSQL (where he led the development of world’s first object-relational database system) and also Cyber Database Solutions in the US. Before founding UniSQL, he worked as a researcher at IBM Almaden Research Center; and as a research director at MCC(Microelectronics and Computer Technology Corporation), where he led a team that developed the ORION object-oriented database system (one of the first OODBs).
He received a Ph.D. in computer science from the University of Illinois at Urbana-Champaign.
In 2017, he received an Order of Service Merit Medal from the Government of Korea for his services to IT industry for both the US and Korea. In 2018, he received an Alumni Outstanding Educator Award from the Computer Science Department of the University of Illinois at Urbana-Champaign.
In the US, he served as Chair of ACM(Association of Computing Machinery) SIGMOD (special interest group on management of data) and founding Chair of ACM SIGKDD (special interest group on knowledge discovery and data mining). He also served as Editor-in-Chief of ACM Transactions on Database Systems, and founding Editor-in-Chief of ACM Transactions on Internet Technology
Machine Learning in Healthcare
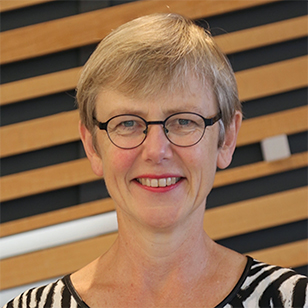
Gill Dobbie
Professor of School of Computer Science, University of Auckland,
New Zealand
Abstract:
My vision is that, through the application of machine learning, our research will be better positioned to help solve some of the major global issues facing the world today and in the future. We are currently on a journey as a community, developing a myriad of novel methods for solving problems but there is still a translational gap between the development of novel methods and their application in society.
The term machine learning was coined in 1959, over 60 years ago, and the number of publications in the area is increasing substantially every year with over 100,000 in 2021 alone. We are developing new methods that are smarter, faster and more accurate, but what impact are we having outside our own research community?
Many of us demonstrate that our proposed method’s accuracy is better than a benchmark on synthetic data sets under various conditions with different parameter settings, and then show that the method also performs well in the real world in a case study on real world data sets. How do we bridge the gulf between our research community and the communities where we could make a real difference?
We have been working towards taking the next step, working with healthcare experts to understand their problems and where our research can have an impact in their domain.
In this keynote, I will discuss some of our projects in the area, and highlight lessons learned and challenges.
Bio:
Gill has a wide range of research interests across artificial intelligence, data management, and software engineering, with a focus on how software systems can be designed to be fast, safe and accurate.
Her research has been applied across a range of domains from healthcare to the environment. Her recent work in data stream mining and concept drift has been used to monitor the accuracy of medical linear accelerators, used in Radiography Departments to treat cancer patients, and freshwater sensors that monitor the quality of the water.
She has also worked in the foundations of database systems, defining logical models for various kinds of database systems, and reasoning about the correctness of algorithms in that setting. With colleagues at the National University of Singapore, she defined a data model for semistructured data (called ORA-SS), providing a language independent description of the data. With this group she has used the ORA-SS data model to define a normal form for ORA-SS schema, defined valid views for semistructured databases, and described a storage structure for semistructured databases using object relational databases.
Gill is on the boards of Precision Driven Health and PlantTech and chairs a number of committees including the Plant and Food Science Advisory Panel and AI Researchers Association.